What’s Changed Since Last Time?
Updated Group MK table for easier comparison between groups
Age Group ANOVA for all metrics
Age Group ANOVA for outlier years
Age Groups
Group 1: Aquistion prior to 1896
Group 2: Acquisition between 1897 and 1909
Group 3: Acquisition between 1910 and 1957
Group 4: Acquisition between 1958 and 1990
Age Group Mann Kendall Analysis
Metrics included in this table were selected because at least one group showed a significant trend for that metric. Excluded metrics showed no trends for any group.
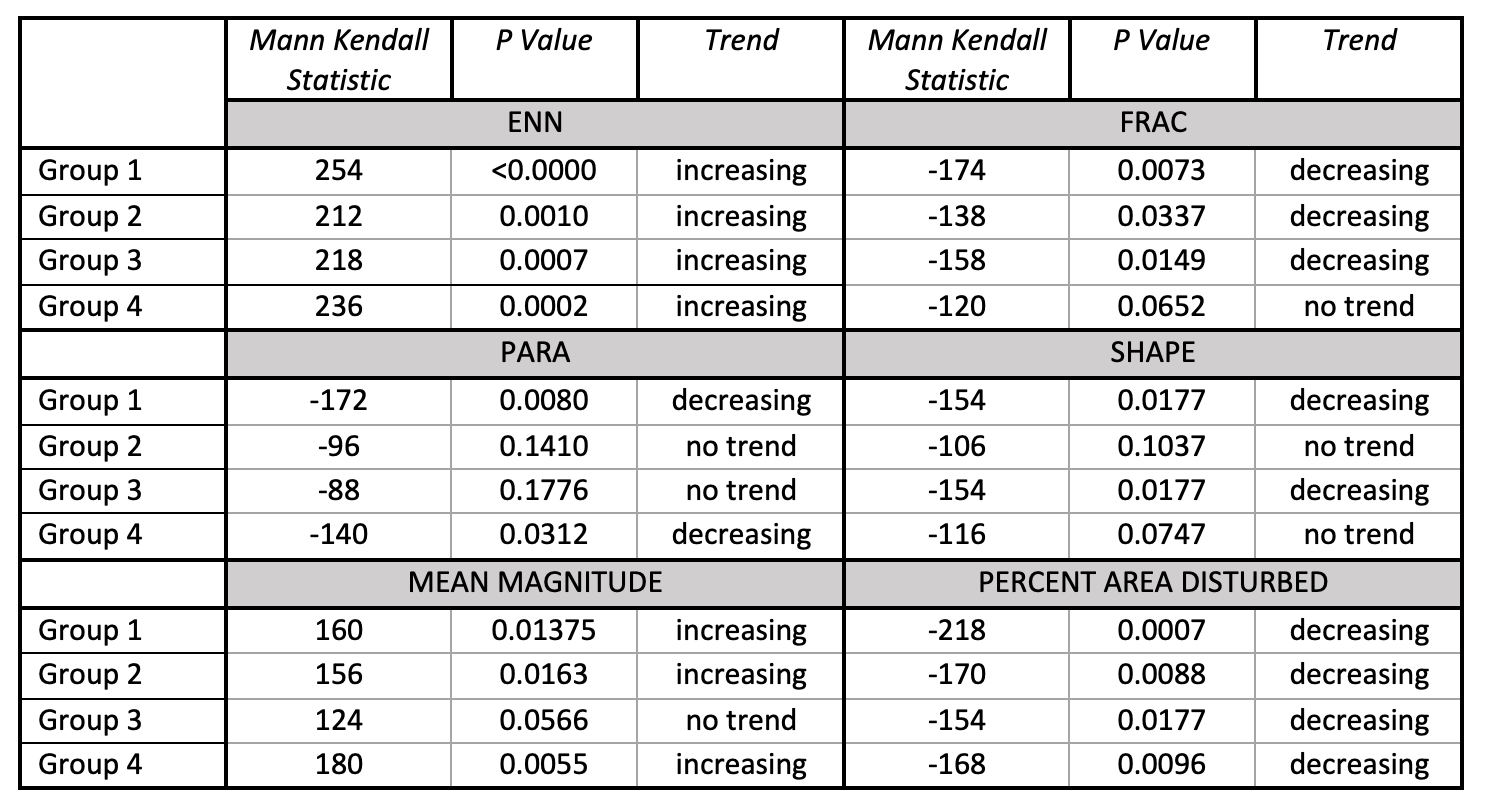
Metric Age Group ANOVAs
ANOVA for metric values by age group. Outliers are hidden for all plots (except core area metrics) for clarity.
Area
Analysis of Variance Table
Response: area
Df Sum Sq Mean Sq F value Pr(>F)
bin 3 594 197.834 3.5435 0.0139 *
Residuals 116212 6488204 55.831
---
Signif. codes: 0 '***' 0.001 '**' 0.01 '*' 0.05 '.' 0.1 ' ' 1
Tukey multiple comparisons of means
95% family-wise confidence level
Fit: aov(formula = model)
$`grouped_patches$bin`
diff lwr upr p adj
2-1 0.07444524 -0.08368595 0.2325764 0.6208127
3-1 0.16925322 0.02583420 0.3126722 0.0129896
4-1 0.13861152 -0.02598488 0.3032079 0.1335367
3-2 0.09480797 -0.07642107 0.2660370 0.4852139
4-2 0.06416628 -0.12515447 0.2534870 0.8200148
4-3 -0.03064169 -0.20785876 0.1465754 0.9707479
CAI
CAI is the patch core area index, which is equal to the percentage of the patch that is not edge. For the vast majority of the patches analyzed here, all cells are edge cells (core = 0) so this metric is hugely zero inflated. This pattern is visible in all the core area metrics.
Analysis of Variance Table
Response: cai
Df Sum Sq Mean Sq F value Pr(>F)
bin 3 1611 537.01 29.178 < 2.2e-16 ***
Residuals 116212 2138857 18.40
---
Signif. codes: 0 '***' 0.001 '**' 0.01 '*' 0.05 '.' 0.1 ' ' 1
Tukey multiple comparisons of means
95% family-wise confidence level
Fit: aov(formula = model)
$`grouped_patches$bin`
diff lwr upr p adj
2-1 0.17061017 0.07981848 0.26140187 0.0000082
3-1 0.28635885 0.20401421 0.36870348 0.0000000
4-1 0.19910977 0.10460604 0.29361350 0.0000004
3-2 0.11574867 0.01743679 0.21406056 0.0132852
4-2 0.02849960 -0.08019972 0.13719891 0.9071224
4-3 -0.08724908 -0.18899901 0.01450086 0.1223891
Circle
Analysis of Variance Table
Response: circle
Df Sum Sq Mean Sq F value Pr(>F)
bin 3 0.03 0.0090487 1.0082 0.3878
Residuals 116212 1042.98 0.0089748
Tukey multiple comparisons of means
95% family-wise confidence level
Fit: aov(formula = model)
$`grouped_patches$bin`
diff lwr upr p adj
2-1 -0.0005535903 -0.002558496 0.0014513153 0.8934363
3-1 -0.0006984824 -0.002516856 0.0011198912 0.7569754
4-1 -0.0013666959 -0.003453572 0.0007201805 0.3329347
3-2 -0.0001448921 -0.002315862 0.0020260778 0.9982094
4-2 -0.0008131056 -0.003213456 0.0015872446 0.8202562
4-3 -0.0006682135 -0.002915104 0.0015786771 0.8706448
Contig
Analysis of Variance Table
Response: contig
Df Sum Sq Mean Sq F value Pr(>F)
bin 3 0.75 0.251244 20.754 1.946e-13 ***
Residuals 116212 1406.82 0.012106
---
Signif. codes: 0 '***' 0.001 '**' 0.01 '*' 0.05 '.' 0.1 ' ' 1
Tukey multiple comparisons of means
95% family-wise confidence level
Fit: aov(formula = model)
$`grouped_patches$bin`
diff lwr upr p adj
2-1 0.0039908080 0.0016623169 0.0063192990 0.0000630
3-1 0.0063173298 0.0042054764 0.0084291832 0.0000000
4-1 0.0030854018 0.0006617101 0.0055090935 0.0059165
3-2 0.0023265218 -0.0001948358 0.0048478795 0.0828128
4-2 -0.0009054062 -0.0036931654 0.0018823530 0.8381254
4-3 -0.0032319280 -0.0058414597 -0.0006223963 0.0079808
Core
Like CAI above, this metric measures the total area that is core area in a patch (in ha). For the vast majority of the patches analyzed here, all cells are edge cells (core = 0) so this metric is hugely zero inflated.
0% 25% 50% 75% 100%
0.00 0.00 0.00 0.00 367.38
Analysis of Variance Table
Response: core
Df Sum Sq Mean Sq F value Pr(>F)
bin 3 66 21.9900 3.2584 0.02058 *
Residuals 116212 784285 6.7487
---
Signif. codes: 0 '***' 0.001 '**' 0.01 '*' 0.05 '.' 0.1 ' ' 1
Tukey multiple comparisons of means
95% family-wise confidence level
Fit: aov(formula = model)
$`grouped_patches$bin`
diff lwr upr p adj
2-1 0.025762257 -0.029216178 0.08074069 0.6243997
3-1 0.053619162 0.003755799 0.10348253 0.0292910
4-1 0.051750584 -0.005475652 0.10897682 0.0927695
3-2 0.027856906 -0.031675339 0.08738915 0.6254763
4-2 0.025988328 -0.039833973 0.09181063 0.7410528
4-3 -0.001868578 -0.063482715 0.05974556 0.9998305
ENN
Analysis of Variance Table
Response: enn
Df Sum Sq Mean Sq F value Pr(>F)
bin 3 659339 219780 13.036 1.651e-08 ***
Residuals 116212 1959205350 16859
---
Signif. codes: 0 '***' 0.001 '**' 0.01 '*' 0.05 '.' 0.1 ' ' 1
Tukey multiple comparisons of means
95% family-wise confidence level
Fit: aov(formula = model)
$`grouped_patches$bin`
diff lwr upr p adj
2-1 -0.05050715 -2.798371 2.697357 0.9999622
3-1 0.71483404 -1.777375 3.207043 0.8822446
4-1 6.53703195 3.676821 9.397243 0.0000000
3-2 0.76534119 -2.210126 3.740808 0.9117630
4-2 6.58753910 3.297690 9.877388 0.0000016
4-3 5.82219791 2.742676 8.901720 0.0000071
Frac
Analysis of Variance Table
Response: enn
Df Sum Sq Mean Sq F value Pr(>F)
bin 3 659339 219780 13.036 1.651e-08 ***
Residuals 116212 1959205350 16859
---
Signif. codes: 0 '***' 0.001 '**' 0.01 '*' 0.05 '.' 0.1 ' ' 1
Tukey multiple comparisons of means
95% family-wise confidence level
Fit: aov(formula = model)
$`grouped_patches$bin`
diff lwr upr p adj
2-1 -0.05050715 -2.798371 2.697357 0.9999622
3-1 0.71483404 -1.777375 3.207043 0.8822446
4-1 6.53703195 3.676821 9.397243 0.0000000
3-2 0.76534119 -2.210126 3.740808 0.9117630
4-2 6.58753910 3.297690 9.877388 0.0000016
4-3 5.82219791 2.742676 8.901720 0.0000071
Gyrate
Analysis of Variance Table
Response: gyrate
Df Sum Sq Mean Sq F value Pr(>F)
bin 3 34662 11554.1 12.284 4.96e-08 ***
Residuals 116212 109307999 940.6
---
Signif. codes: 0 '***' 0.001 '**' 0.01 '*' 0.05 '.' 0.1 ' ' 1
Tukey multiple comparisons of means
95% family-wise confidence level
Fit: aov(formula = model)
$`grouped_patches$bin`
diff lwr upr p adj
2-1 0.5676259 -0.08142868 1.21668058 0.1108563
3-1 1.3806704 0.79200236 1.96933841 0.0000000
4-1 0.7271648 0.05157353 1.40275617 0.0290721
3-2 0.8130444 0.11022926 1.51585963 0.0156693
4-2 0.1595389 -0.61753431 0.93661211 0.9524634
4-3 -0.6535055 -1.38089877 0.07388769 0.0961511
Ncore
The third core area metric, ncore represents the number of core areas in the patch. As in the other core area metrics this one is again heavily zero inflated.
Analysis of Variance Table
Response: ncore
Df Sum Sq Mean Sq F value Pr(>F)
bin 3 83 27.6303 4.8755 0.002166 **
Residuals 116212 658590 5.6671
---
Signif. codes: 0 '***' 0.001 '**' 0.01 '*' 0.05 '.' 0.1 ' ' 1
Tukey multiple comparisons of means
95% family-wise confidence level
Fit: aov(formula = model)
$`grouped_patches$bin`
diff lwr upr p adj
2-1 0.03173715 -0.01864339 0.08211769 0.3681629
3-1 0.06427195 0.01857871 0.10996520 0.0017177
4-1 0.04935038 -0.00308997 0.10179074 0.0737622
3-2 0.03253480 -0.02201871 0.08708831 0.4181599
4-2 0.01761324 -0.04270429 0.07793076 0.8766255
4-3 -0.01492157 -0.07138286 0.04153972 0.9051306
Para
Analysis of Variance Table
Response: para
Df Sum Sq Mean Sq F value Pr(>F)
bin 3 0.016 0.0054958 15.854 2.65e-10 ***
Residuals 116212 40.284 0.0003466
---
Signif. codes: 0 '***' 0.001 '**' 0.01 '*' 0.05 '.' 0.1 ' ' 1
Tukey multiple comparisons of means
95% family-wise confidence level
Fit: aov(formula = model)
$`grouped_patches$bin`
diff lwr upr p adj
2-1 -0.0005666264 -9.606495e-04 -1.726033e-04 0.0012609
3-1 -0.0009402585 -1.297623e-03 -5.828944e-04 0.0000000
4-1 -0.0004350295 -8.451623e-04 -2.489669e-05 0.0326008
3-2 -0.0003736321 -8.002917e-04 5.302758e-05 0.1100960
4-2 0.0001315970 -3.401427e-04 6.033366e-04 0.8905214
4-3 0.0005052290 6.364872e-05 9.468093e-04 0.0173283
Perim
Analysis of Variance Table
Response: perim
Df Sum Sq Mean Sq F value Pr(>F)
bin 3 8.5868e+07 28622719 3.6011 0.01284 *
Residuals 116212 9.2368e+11 7948237
---
Signif. codes: 0 '***' 0.001 '**' 0.01 '*' 0.05 '.' 0.1 ' ' 1
Tukey multiple comparisons of means
95% family-wise confidence level
Fit: aov(formula = model)
$`grouped_patches$bin`
diff lwr upr p adj
2-1 27.15467 -32.50985 86.81920 0.6463319
3-1 66.21793 12.10447 120.33140 0.0090522
4-1 47.62941 -14.47451 109.73332 0.1992754
3-2 39.06326 -25.54322 103.66974 0.4056180
4-2 20.47473 -50.95794 91.90740 0.8824564
4-3 -18.58853 -85.45435 48.27729 0.8915138
Shape
Analysis of Variance Table
Response: shape
Df Sum Sq Mean Sq F value Pr(>F)
bin 3 4 1.31650 3.8112 0.009601 **
Residuals 116212 40143 0.34543
---
Signif. codes: 0 '***' 0.001 '**' 0.01 '*' 0.05 '.' 0.1 ' ' 1
Tukey multiple comparisons of means
95% family-wise confidence level
Fit: aov(formula = model)
$`grouped_patches$bin`
diff lwr upr p adj
2-1 0.005749251 -0.006689051 0.018187554 0.6347910
3-1 0.014630164 0.003349094 0.025911234 0.0047871
4-1 0.003106984 -0.009839860 0.016053828 0.9268550
3-2 0.008880913 -0.004587642 0.022349467 0.3268006
4-2 -0.002642267 -0.017533882 0.012249347 0.9685108
4-3 -0.011523180 -0.025462741 0.002416381 0.1455298
Mean Magnitude
Analysis of Variance Table
Response: mean_mag
Df Sum Sq Mean Sq F value Pr(>F)
bin 3 888340 296113 131.97 < 2.2e-16 ***
Residuals 116212 260763322 2244
---
Signif. codes: 0 '***' 0.001 '**' 0.01 '*' 0.05 '.' 0.1 ' ' 1
Tukey multiple comparisons of means
95% family-wise confidence level
Fit: aov(formula = model)
$`grouped_patches$bin`
diff lwr upr p adj
2-1 2.078648 1.0761612 3.081134 0.0000006
3-1 3.513163 2.6039452 4.422380 0.0000000
4-1 7.926428 6.8829549 8.969902 0.0000000
3-2 1.434515 0.3489934 2.520036 0.0038312
4-2 5.847781 4.6475651 7.047996 0.0000000
4-3 4.413266 3.2897826 5.536749 0.0000000
Summary
With the exception of the circle metric, at least one age group is significantly different for all metrics. The mean disturbance magnitude is the only metric where each age group was significantly different from all other groups. There are no clear patterns within metric types (shape, area/edge, core area).
Outlier Year ANOVAs
ANOVA by age groups for years with outlier values in specific metrics. Outliers again removed for clarity.
Mean Disturbance Magnitude Outliers
1995
Analysis of Variance Table
Response: mean_mag
Df Sum Sq Mean Sq F value Pr(>F)
bin 3 245013 81671 36.157 < 2.2e-16 ***
Residuals 6728 15196959 2259
---
Signif. codes: 0 '***' 0.001 '**' 0.01 '*' 0.05 '.' 0.1 ' ' 1
Tukey multiple comparisons of means
95% family-wise confidence level
Fit: aov(formula = model)
$`year_patches$bin`
diff lwr upr p adj
2-1 -1.222921 -5.6883643 3.242523 0.8956460
3-1 9.355151 5.4284343 13.281867 0.0000000
4-1 13.553241 9.3584579 17.748024 0.0000000
3-2 10.578071 6.2165457 14.939597 0.0000000
4-2 14.776162 10.1718149 19.380509 0.0000000
4-3 4.198091 0.1141069 8.282074 0.0412098
2007
Analysis of Variance Table
Response: mean_mag
Df Sum Sq Mean Sq F value Pr(>F)
bin 3 114106 38035 6.1644 0.0003804 ***
Residuals 851 5250829 6170
---
Signif. codes: 0 '***' 0.001 '**' 0.01 '*' 0.05 '.' 0.1 ' ' 1
Tukey multiple comparisons of means
95% family-wise confidence level
Fit: aov(formula = model)
$`year_patches$bin`
diff lwr upr p adj
2-1 11.603668 -8.420391 31.627728 0.4429639
3-1 -20.682260 -37.912994 -3.451526 0.0111088
4-1 -2.100984 -22.527012 18.325045 0.9935008
3-2 -32.285929 -52.864351 -11.707506 0.0003411
4-2 -13.704652 -37.024085 9.614781 0.4302110
4-3 18.581277 -2.388491 39.551045 0.1032808
2021
Analysis of Variance Table
Response: mean_mag
Df Sum Sq Mean Sq F value Pr(>F)
bin 3 10127 3375.8 1.6595 0.1736
Residuals 3153 6413883 2034.2
Tukey multiple comparisons of means
95% family-wise confidence level
Fit: aov(formula = model)
$`year_patches$bin`
diff lwr upr p adj
2-1 2.604837 -3.216998 8.426673 0.6584312
3-1 3.990706 -1.468656 9.450067 0.2373037
4-1 -0.377839 -6.040349 5.284671 0.9982073
3-2 1.385868 -5.006368 7.778104 0.9445847
4-2 -2.982676 -9.549264 3.583911 0.6474418
4-3 -4.368545 -10.616018 1.878929 0.2747389
Shape Metrics
Only ANOVA that showed significant difference between groups included below. Patch level shape metrics are circle, contig, frac, para and shape.
1995
Contig
Analysis of Variance Table
Response: contig
Df Sum Sq Mean Sq F value Pr(>F)
bin 3 1.32 0.44016 23.39 4.68e-15 ***
Residuals 6728 126.61 0.01882
---
Signif. codes: 0 '***' 0.001 '**' 0.01 '*' 0.05 '.' 0.1 ' ' 1
Tukey multiple comparisons of means
95% family-wise confidence level
Fit: aov(formula = model)
$`year_patches$bin`
diff lwr upr p adj
2-1 0.01727986 0.004391068 0.030168657 0.0032196
3-1 0.03506099 0.023727145 0.046394832 0.0000000
4-1 0.02861038 0.016502802 0.040717955 0.0000000
3-2 0.01778113 0.005192275 0.030369977 0.0016284
4-2 0.01133052 -0.001959201 0.024620233 0.1258257
4-3 -0.00645061 -0.018238381 0.005337161 0.4954447
Frac
Analysis of Variance Table
Response: frac
Df Sum Sq Mean Sq F value Pr(>F)
bin 3 0.1307 0.043581 12.122 6.566e-08 ***
Residuals 6728 24.1888 0.003595
---
Signif. codes: 0 '***' 0.001 '**' 0.01 '*' 0.05 '.' 0.1 ' ' 1
Tukey multiple comparisons of means
95% family-wise confidence level
Fit: aov(formula = model)
$`year_patches$bin`
diff lwr upr p adj
2-1 0.0002250884 -0.005408604 0.005858780 0.9996128
3-1 0.0078818738 0.002927851 0.012835896 0.0002567
4-1 0.0099901582 0.004697937 0.015282379 0.0000075
3-2 0.0076567854 0.002154199 0.013159372 0.0019944
4-2 0.0097650698 0.003956135 0.015574005 0.0000933
4-3 0.0021082844 -0.003044150 0.007260719 0.7190472
Para
Analysis of Variance Table
Response: para
Df Sum Sq Mean Sq F value Pr(>F)
bin 3 0.02216 0.0073852 17.347 3.234e-11 ***
Residuals 6728 2.86440 0.0004257
---
Signif. codes: 0 '***' 0.001 '**' 0.01 '*' 0.05 '.' 0.1 ' ' 1
Tukey multiple comparisons of means
95% family-wise confidence level
Fit: aov(formula = model)
$`year_patches$bin`
diff lwr upr p adj
2-1 -0.002265097 -0.0042037631 -0.0003264316 0.0142990
3-1 -0.004624244 -0.0063290222 -0.0029194662 0.0000000
4-1 -0.003494477 -0.0053156354 -0.0016733177 0.0000050
3-2 -0.002359147 -0.0042526966 -0.0004655971 0.0075033
4-2 -0.001229379 -0.0032283495 0.0007695911 0.3899171
4-3 0.001129768 -0.0006432878 0.0029028231 0.3576104
2021
Contig
Analysis of Variance Table
Response: contig
Df Sum Sq Mean Sq F value Pr(>F)
bin 3 0.277 0.092353 4.5387 0.003548 **
Residuals 1971 40.105 0.020348
---
Signif. codes: 0 '***' 0.001 '**' 0.01 '*' 0.05 '.' 0.1 ' ' 1
Tukey multiple comparisons of means
95% family-wise confidence level
Fit: aov(formula = model)
$`year_patches$bin`
diff lwr upr p adj
2-1 0.024562229 -0.001480462 0.05060492 0.0727458
3-1 0.030464762 0.008895055 0.05203447 0.0016436
4-1 0.021372810 -0.003749130 0.04649475 0.1270190
3-2 0.005902533 -0.017812026 0.02961709 0.9190408
4-2 -0.003189418 -0.030175339 0.02379650 0.9902589
4-3 -0.009091952 -0.031791521 0.01360762 0.7319309
Frac
Analysis of Variance Table
Response: frac
Df Sum Sq Mean Sq F value Pr(>F)
bin 3 0.0419 0.0139602 3.8076 0.009781 **
Residuals 1971 7.2265 0.0036664
---
Signif. codes: 0 '***' 0.001 '**' 0.01 '*' 0.05 '.' 0.1 ' ' 1
Tukey multiple comparisons of means
95% family-wise confidence level
Fit: aov(formula = model)
$`year_patches$bin`
diff lwr upr p adj
2-1 0.004577434 -0.0064772676 0.01563214 0.7111202
3-1 0.010766794 0.0016108018 0.01992279 0.0134855
4-1 0.010997433 0.0003335755 0.02166129 0.0402647
3-2 0.006189360 -0.0038770870 0.01625581 0.3897640
4-2 0.006419999 -0.0050350880 0.01787509 0.4738025
4-3 0.000230639 -0.0094049616 0.00986624 0.9999164
Para
Analysis of Variance Table
Response: para
Df Sum Sq Mean Sq F value Pr(>F)
bin 3 0.00475 0.00158403 3.5268 0.01439 *
Residuals 1971 0.88527 0.00044915
---
Signif. codes: 0 '***' 0.001 '**' 0.01 '*' 0.05 '.' 0.1 ' ' 1
Tukey multiple comparisons of means
95% family-wise confidence level
Fit: aov(formula = model)
$`year_patches$bin`
diff lwr upr p adj
2-1 -0.0035473318 -0.007416532 0.0003218685 0.0858400
3-1 -0.0039062403 -0.007110883 -0.0007015976 0.0094653
4-1 -0.0025256023 -0.006258005 0.0012068008 0.3032574
3-2 -0.0003589084 -0.003882215 0.0031643981 0.9937074
4-2 0.0010217295 -0.002987608 0.0050310669 0.9137279
4-3 0.0013806380 -0.001991870 0.0047531460 0.7183820
Shape
Analysis of Variance Table
Response: shape
Df Sum Sq Mean Sq F value Pr(>F)
bin 3 13.44 4.4793 4.8499 0.002298 **
Residuals 1971 1820.39 0.9236
---
Signif. codes: 0 '***' 0.001 '**' 0.01 '*' 0.05 '.' 0.1 ' ' 1
Tukey multiple comparisons of means
95% family-wise confidence level
Fit: aov(formula = model)
$`year_patches$bin`
diff lwr upr p adj
2-1 0.084028700 -0.09142649 0.2594839 0.6068922
3-1 0.197741965 0.05242222 0.3430617 0.0026894
4-1 0.188389168 0.01913727 0.3576411 0.0220977
3-2 0.113713264 -0.04605681 0.2734833 0.2594581
4-2 0.104360468 -0.07744947 0.2861704 0.4523139
4-3 -0.009352797 -0.16228467 0.1435791 0.9986158